Which Predictive Analytics Models Are Highly Effective in Shaping Business Strategy?
Data Science Spotlight

Which Predictive Analytics Models Are Highly Effective in Shaping Business Strategy?
Ever wondered how top leaders are using predictive analytics to shape their business strategies? Insights from a Founder and Chief Marketing Officer and a CEO reveal the latest trends and tools. Discover how a Churn-Prediction Model can enhance client retention and learn about applying A/B Testing for decision-making. This article compiles eight expert insights to give you a comprehensive view.
- Utilize Churn-Prediction Model for Client Retention
- Leverage Time-Series Forecasting for Project Timelines
- Incorporate Recommendation Systems for User Engagement
- Deploy Gradient-Boosting Model for Customer Insights
- Implement Injury-Prevention Forecasting for Better Care
- Apply Generalized Mixed-Models for Trend Analysis
- Use Generalized Additive Model for Risk Assessment
- Employ A/B Testing for Decision-Making
Utilize Churn-Prediction Model for Client Retention
As far as my experience goes, at Kualitatem, predictive analytics has contributed significantly toward understanding the business direction, especially in understanding the expectations of the client and the deployment of resources. One such model was the churn-prediction model, which learned to look for clients who might leave the organization. We were able to reach out to clients who were becoming disengaged by utilizing this model, which analyzed historical data related to client interaction, timelines of various projects, and client feedback. Implementing this model improved our client retention by 20% in a year, as we put out the fires before they turned into blazes. As a result, this method has made it possible for the company to develop more data-driven strategies and eventually improve client satisfaction and retention.

Leverage Time-Series Forecasting for Project Timelines
At Software House, we used predictive analytics to guide our business strategy by leveraging data to forecast client needs, optimize project timelines, and enhance service offerings. One area where this had a significant impact was in identifying patterns in client behavior, such as predicting which industries or client types were most likely to require specific types of software solutions in the near future. By analyzing historical data—ranging from client inquiries to project completion times and the success metrics of past projects—we were able to create predictive models that informed both our marketing and operational strategies. This approach allowed us to proactively target growing industries with tailored solutions, improving lead generation and sales efficiency.
One predictive model that proved particularly effective was a time-series forecasting model we developed to predict project durations and resource allocations. By analyzing variables such as project scope, complexity, client interaction frequency, and team performance, the model helped us more accurately estimate how long a project would take and the resources it would need. This led to more realistic timelines, better resource management, and improved client satisfaction because we could avoid the common pitfall of underestimating project requirements. This model not only enhanced internal operations but also reinforced trust with clients, who appreciated our transparency and precision in delivering on time and within budget.
Incorporate Recommendation Systems for User Engagement
Using predictive analytics to inform business strategy allows you to anticipate trends, optimize decision-making, and stay ahead of market shifts. The key is integrating data-driven insights into everyday operations to guide choices on everything from product development to marketing campaigns. For business leaders, the best advice is to embrace predictive tools that can forecast customer behavior, allowing you to tailor strategies proactively rather than reactively.
When I started incorporating predictive analytics into the Christian Companion App's marketing, it changed how we approached content generation and user engagement. We analyzed user data and saw patterns in how people interacted with our app. For example, we noticed that users tended to be more engaged with Bible study content during particular times of the week, so we adjusted our push notifications and marketing campaigns to match those behaviors. This led to a significant increase in user interaction and retention.
The most effective model we used was a recommendation system powered by machine learning. This model analyzed user behavior to predict which types of content—whether text, images, or videos—would be most engaging for each individual. It allowed us to personalize the experience on a granular level, making our marketing more efficient and boosting user satisfaction. The strategy was simple: use the data we already had to forecast trends and then act on those forecasts to improve everything from product offerings to customer outreach.
Predictive analytics isn't just about the numbers; it's about understanding what drives your audience and leveraging those insights to stay one step ahead. In our case, by personalizing user experience through predictive models, we saw improved engagement and loyalty. This evidence underscores the power of AI-driven strategies in today's business world—those who don't leverage these tools risk falling behind.
Deploy Gradient-Boosting Model for Customer Insights
At RecurPost, predictive analytics helped us stay a step ahead by showing which customers might be drifting away. Using a tool called a gradient-boosting model, we could spot patterns in how people interacted with our service, giving us early signs of who might leave. This let us reach out personally and make changes that mattered to them, which helped us build stronger, lasting connections.
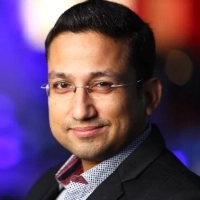
Implement Injury-Prevention Forecasting for Better Care
In recent years, I integrated predictive analytics into The Alignment Studio’s operations to improve patient outcomes and streamline resource allocation. With over 30 years in physiotherapy, I have seen firsthand how patterns in patient demographics, injuries, and rehabilitation progress can reveal trends that are invaluable for proactive decision-making. Using my experience in musculoskeletal and sports injuries, I developed models that analyze historical patient data to predict busy seasons and common injury types, especially among Melbourne’s active sports communities. This allowed us to prepare our team’s schedules, allocate resources, and adapt our services to better meet demand, significantly enhancing both patient care and operational efficiency.
One particularly effective model was our injury-prevention forecasting tool, which helped us identify recurring patterns in postural and repetitive-strain injuries among office-based patients. This tool not only improved our intake protocols but also influenced our strategy to expand workplace wellness programs. As we saw a rise in these injuries, likely due to the shift toward hybrid work environments, the model validated the need to offer services like ergonomic assessments and targeted Pilates classes. By anticipating these needs, we were able to increase client satisfaction and maintain a proactive stance on injury prevention, ultimately supporting better long-term health outcomes for our clients.
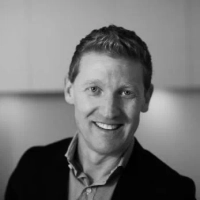
Apply Generalized Mixed-Models for Trend Analysis
We utilized predictive analytics as a powerful method to inform our business strategy. The historical data in our warehouse enabled us to forecast future trends effectively. While there isn't a single model that can comprehensively address all situations, we found that Generalized Mixed-Models and Bayesian-Models are the two most efficient types we employed.
Generalized Mixed-Models account for both fixed and random variables, making them particularly useful for analyzing historical and longitudinal data. On the other hand, Bayesian-Models excel in predicting posterior probabilities of an event, especially when prior information or probabilities are available. These models have proven to be stronger in scenarios where previous insights can guide our predictions.
Use Generalized Additive Model for Risk Assessment
I recently worked with a fundamental statistical model to fit nonlinear relationships in GIS data called "GAM" (Generalized Additive Model), and it helped me bifurcate and assess property risk (choropleth map) based on certain climatological and topographical signals, further helping to strategize and prioritize business in insurance.
Employ A/B Testing for Decision-Making
A/B testing is the crème de la crème of data-scientific modeling to help steer decision-making. This methodology is the true litmus test of a data scientist's ability because it tests the limitations of condensing academic jargon into insights digestible by decision-makers. The art of the A/B test is in the explanation, and it truly is an art. Explaining odds ratios, confidence intervals, and p-values is elementary when talking to scientists and people with a statistics background, but it demands deep understanding when presenting to a layperson.
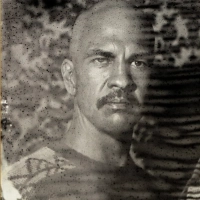