Which Metrics Are Crucial for Evaluating Machine Learning Models in Production for a Data Scientist?
Data Science Spotlight

Which Metrics Are Crucial for Evaluating Machine Learning Models in Production for a Data Scientist?
Imagine unlocking possible success by tracking a pivotal metric in your machine learning models that you might be overlooking. Insights from a Business Development Manager and a CEO illuminate new perspectives in this comprehensive discussion. Starting with monitoring conversion rates to gauge effectiveness and concluding with performance analysis through confusion matrix reviews, the conversation covers four impactful insights. Explore this discourse to uncover techniques for measuring machine learning model success in production.
- Monitor Conversion Rate for Effectiveness
- Utilize F1 Score for Balanced Evaluation
- Measure Success with Accuracy
- Analyze Performance Using Confusion Matrix
Monitor Conversion Rate for Effectiveness
The "Conversion Rate" is a key metric for evaluating machine-learning model success, representing the percentage of users who perform a desired action, like making a purchase. Influenced by factors such as user behavior, demographics, and personalized offers, a high conversion rate indicates the model's effectiveness in identifying likely responders, thereby validating its performance. It reflects the model's ability to optimize user engagement from targeted offerings.
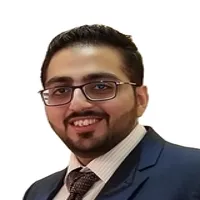
Utilize F1 Score for Balanced Evaluation
A specific metric I often use to measure the success of machine-learning models in production is the F1 score. This metric provides a balance between precision and recall, making it particularly valuable for classification problems where the distribution of classes is imbalanced. The F1 score helps ensure that the model not only identifies true positives effectively but also minimizes false positives and false negatives, which is critical in applications such as fraud detection or medical diagnosis.
Additionally, I complement the F1 score with metrics like accuracy, ROC-AUC, and confusion matrices to gain a comprehensive understanding of the model's performance. This multifaceted approach allows for better insights into how well the model operates in real-world scenarios, enabling continuous improvement and refinement based on user feedback and performance data. Regularly monitoring these metrics helps us adapt to changing data patterns and maintain the effectiveness of our machine-learning solutions.
Measure Success with Accuracy
One specific metric we frequently use to measure the success of our machine-learning models in production is accuracy. It allows us to evaluate how well the model is predicting outcomes correctly, ensuring it meets performance expectations and delivers reliable insights for our operations. Additionally, we track precision and recall to maintain a balance between true positives and false positives, which is critical for optimizing our automation systems at Advanced Motion Controls.
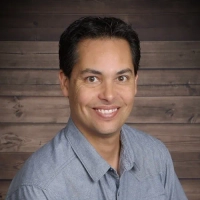
Analyze Performance Using Confusion Matrix
One metric I often use to evaluate machine-learning models in production is the confusion matrix. It's like the scoreboard of your model's predictions, breaking them down into wins and losses: true positives, true negatives, false positives, and false negatives. From this, you can calculate key stats like precision, recall, and the F1-score—all crucial to know whether your model is hitting the mark or needs a bit of adjustment. It's a great tool to identify where your model is doing well and where it might need some extra attention, helping you make data-driven tweaks for better performance.
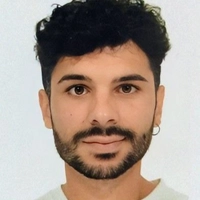