What Validation Techniques Ensure the Accuracy of Data Analysis Results?
Data Science Spotlight

What Validation Techniques Ensure the Accuracy of Data Analysis Results?
Picture a dance where only those with the most precise steps make it to the spotlight. In the competitive realm of data-driven industries, it's the methodical moves made by professionals like CEOs and Founders that set the tone for success. This blog post uncovers essential insights, starting with employing cross-validation techniques and concluding with cross-checking with independent sources, with a total of four expert strategies that live up to their ethos, validating analysis with accuracy.
- Employ Cross-Validation Techniques
- Investigate Unexpected Findings
- Consult With Data Sources
- Cross-Check With Independent Sources
Employ Cross-Validation Techniques
There was a project at Software House where we analyzed user engagement data from our mobile applications to identify trends that could inform future development. Given the importance of the findings, it was crucial to validate the results to ensure accuracy before making any strategic decisions.
One effective approach we employed was cross-validation through multiple data sources. We compared our initial analysis with data from alternative sources, such as user feedback surveys and industry benchmarks. By aggregating insights from different datasets, we could identify any discrepancies and ensure that our conclusions were consistent across multiple channels.
Additionally, we engaged team members from different departments to review the findings, as they brought unique perspectives and insights that could uncover potential biases or overlooked factors. This collaborative review process not only enhanced the accuracy of our analysis but also fostered a sense of ownership among team members regarding the decisions made based on that data. Ultimately, this thorough validation process gave us the confidence to move forward with our strategies, knowing they were grounded in accurate and reliable data.
Investigate Unexpected Findings
In a recent project, we were analyzing customer-engagement metrics across multiple social media platforms for Luxury Fire. The goal was to understand which platform drove the most conversions for our high-end fireplaces. After the initial analysis, we noticed unusually high engagement on a particular platform that didn't align with our historical data.
To validate these results, I took a two-step approach. First, I cross-checked the raw data by running the same queries through different analytics tools to ensure consistency. Then, I conducted a manual review of the data points, checking for any outliers or anomalies that might have skewed the results. During this process, I discovered a tagging issue in our social-media campaigns, where unrelated posts were being counted as conversions.
This approach, using multiple tools and manually reviewing the data, helped us pinpoint the error and correct our strategy. For any business dealing with data analysis, it's crucial to verify results with more than one method and always investigate unexpected findings.
Consult With Data Sources
Validating data can be a difficult task. When working at a data-analytics firm a few years ago, I was tasked with creating an automated sales-forecasting application that pulled data from relevant internal sources. This data was then pulled into a front-end report that needed to be validated. I sat with leaders in the field responsible for the results of the report and looked at examples in their organization and how they were reflecting on the report. Moral of the story is, don't discount actually sitting down with the people who you are sourcing the data from. They can be invaluable in pointing out inaccuracies that you, as a data validator, may not know. Good data sleuthing at the beginning will save you from a world of hurt down the road.
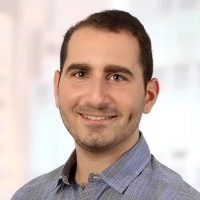
Cross-Check With Independent Sources
One approach I often rely on to validate the results of a data analysis is cross-checking with independent data sources. In one project, we were analyzing operational costs for a client, and the initial findings showed unusually low figures in certain categories. To ensure accuracy, we validated the results by comparing them with external industry benchmarks and internal financial records. This helped confirm the integrity of the data and revealed a discrepancy in one dataset due to outdated entries. By triangulating multiple sources, we corrected the data and ensured our analysis was accurate and reliable.
