What Custom Metrics Have You Developed for a Project?
Data Science Spotlight

What Custom Metrics Have You Developed for a Project?
Exploring the innovative ways professionals enhance model evaluation, we’ve gathered insights from a Data Scientist and a Senior Research Scientist. While they share their specialized metrics, we’ve also included additional answers to provide a broader perspective. From developing a relative-sentiment metric for benchmarking to evaluating supply chain risk, discover the diverse approaches to custom metric development.
- Develop Relative-Sentiment Metric for Benchmarking
- Penalize Under-Forecasting in Delivery Models
- Use High-Value Customer Precision Score
- Measure Social Media Engagement Efficiency
- Visualize Product Feature Adoption
- Identify Anomalous User Interactions
- Evaluate Supply Chain Risk
Develop Relative-Sentiment Metric for Benchmarking
I worked on a sentiment-analysis project for a client, analyzing social media posts related to a topic that no one really posts about in a positive way. Initially, the sentiment scores were overwhelmingly negative, which wasn't very helpful.
However, when I compared these sentiments to those of competitors, I found that all were negative, but some competitors were more negative than others. I developed a relative-sentiment metric to benchmark my client against others, showing they ranked in the top 10 out of 40 companies. By reframing the problem and developing this new metric, we were able to provide a more meaningful evaluation and highlight actionable feedback from competitors with higher scores, leading to strategic improvements for my client.
Penalize Under-Forecasting in Delivery Models
I was developing a forecast model for middle-mile and last-mile delivery operations for one of the largest B2B retailers in the US. Typically, the default method for training the model parameters is to minimize the OLS (ordinary least squares) loss function or some standard loss function. Even though this will give us the best model by equally penalizing under-forecasting and over-forecasting, the forecast generated from such a model might not always align with operational challenges and bottlenecks.
For example, in my use case, over-forecasting was preferred over under-forecasting for delivery operations. It is not easy to arrange extra delivery capacity on short notice, which leads to delays in fulfilling customer orders. Therefore, the delivery managers wanted to get forecasts that allow them to fulfill all customer orders in a timely manner rather than planning for less capacity and losing out on customer satisfaction. I changed the loss function to penalize under-forecasting more so that the model parameters could be trained accordingly. This model generated a more robust forecast from a delivery operations perspective.
The takeaway is understanding the use case for any mathematical model and designing the metrics accordingly to suit the context.
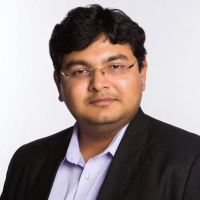
Use High-Value Customer Precision Score
In one of my recent projects, I came up with a custom metric called the 'High-Value Customer Precision Score' (HV-CPS) to evaluate our predictive model for forecasting customer lifetime value. Instead of just relying on the usual metrics like Mean Absolute Error (MAE) or Root Mean Squared Error (RMSE), which give a general sense of accuracy, I wanted something that specifically focused on our most valuable customers.
The HV-CPS metric zeroed in on how well we predicted our high-value customers. It gave more weight to accurately identifying these top-tier customers and penalized the model more for false positives. This way, we ensured that our model's performance was directly tied to our business goals, particularly in terms of prioritizing marketing and customer retention efforts.
Using the HV-CPS metric made a big difference. It helped us pinpoint where the model was doing well and where it needed improvement, allowing us to fine-tune our features and algorithms more effectively. As a result, our accuracy in identifying high-value customers improved significantly. This led to more effective and targeted marketing strategies, better customer retention, and ultimately, higher revenue growth. It was rewarding to see how a custom metric could translate into such tangible business benefits.
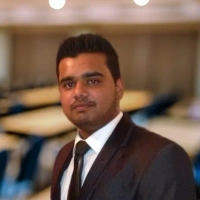
Measure Social Media Engagement Efficiency
Social media engagement efficiency index measures how effectively a brand engages its audience on social platforms. By evaluating likes, comments, and shares relative to posts and followers, companies gain insights into content effectiveness. This metric can guide social media strategies to increase audience interaction.
It plays a crucial role in boosting brand presence and customer engagement. Start tracking this index to enhance your social media impact.
Visualize Product Feature Adoption
Product feature adoption heatmap visualizes which features of a product are being most used by customers. Evaluating usage patterns helps businesses understand what features resonate with users and which do not. This data-driven insight allows for better product development and optimization.
By focusing on popular features, companies can improve user satisfaction and engagement. Implement this heatmap to refine your product offerings and ensure they meet customer needs.
Identify Anomalous User Interactions
User interaction anomaly detection rate identifies unusual user behaviors that could indicate fraud or technical issues. Monitoring this metric helps maintain the integrity of a platform and ensures a secure user experience. Detecting anomalies early can prevent potential problems and improve overall system reliability.
This can be crucial for maintaining trust and safety among your user base. Make use of this metric to protect and enhance user interactions on your platform.
Evaluate Supply Chain Risk
Supply chain risk assessment score evaluates the potential risks within a supply chain process. By analyzing factors such as delivery times, supplier reliability, and geopolitical factors, companies can identify vulnerabilities. This metric helps in planning for disruptions and maintaining smooth operations.
Proactively managing these risks ensures consistent product availability and service quality. Adopt this score system to safeguard your supply chain and business continuity.