What Are Examples of Using Outlier Detection to Enhance Analysis Quality?
Data Science Spotlight

What Are Examples of Using Outlier Detection to Enhance Analysis Quality?
Ever wondered how outlier detection can significantly enhance the quality of data analyses? This blog post explores six compelling insights, starting with improving audit selection accuracy and concluding with pinpointing unusual data patterns. Readers will discover how these techniques ensure reliable data models, enhance forecasting accuracy, increase statistical analysis precision, and reveal unusual customer behaviors. Dive into these practical examples to understand the transformative power of outlier detection in data analysis.
- Improve Audit Selection Accuracy
- Ensure Reliable Data Models
- Enhance Forecasting Accuracy
- Increase Statistical Analysis Precision
- Reveal Unusual Customer Behaviors
- Pinpoint Unusual Data Patterns
Improve Audit Selection Accuracy
At RSI, there are a variety of applications of outlier detection (or anomaly detection). In our analytics work, these applications include fraud scoring, collections analysis, audit selection, and others.
In the audit-selection framework, given the right feature data, machine learning (ML) can be a powerful tool for assisting in the selection of potentially productive audits (which at least pay for the auditor's time). However, training an accurate supervised ML model relies on having a sufficient number of labeled examples in the dataset. In the case of audits, a single labeled example can take an auditor anywhere from a few days to a few weeks to provide the change status (underpaid, no change, or overpaid) and the amount due. This audit result can be appealed, etc., and so the time involved to obtain this labeled example can become inordinate. Thus, even with ten years of data, the number of labeled examples in the dataset may be insufficient for a typical supervised ML model training. Thus, we need to use features of the unlabeled dataset to provide more information to the supervised model-training process. This can be done using clustering techniques to find general "behavioral groups" as well as outliers. These unlabeled data "derived features" are incorporated as a part of the supervised audit model. In a particular effort, the RSI analytics team found that including such clustering and anomaly features in the supervised process improved our accuracy in classifying a potentially productive audit from around 65% to about 95%. At RSI, we continue to apply unsupervised approaches to other application areas where labeled data is sparse but where there is still structural information in the unlabeled data, like clusters and anomalies.
We are also applying outlier/anomaly detection as a secondary model to assist in identifying fraud. In this case, there is sufficient data, but as fraudulent behaviors change, the supervised models will suffer from performance drift. Our anomaly detection model assists in identifying fraud outside of the behaviors seen during supervised training, as well as being an indicator that the primary supervised model needs to be tuned and/or include newer data in the training process.
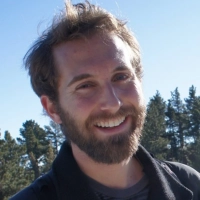
Ensure Reliable Data Models
Outlier detection is crucial for identifying data points that can distort statistical models, leading to more accurate analyses. By pinpointing these aberrant values, analysts can refine their datasets to reflect true patterns. This process helps ensure the models' outputs are reliable and valid. Enhanced accuracy in data models supports better decision-making.
Therefore, implementing outlier detection is essential for maintaining high-quality analysis. Start incorporating outlier detection in data analysis workflows today to achieve reliable results. Ensure your team is trained in these techniques to maximize the benefits.
Enhance Forecasting Accuracy
Detecting anomalies in time-series data can significantly improve forecasting accuracy. By identifying outliers, one can adjust the forecast models to account for unusual spikes or drops in the data. This leads to more reliable predictions and better planning for future events. Enhanced forecasting helps businesses allocate resources more efficiently and anticipate changes more effectively.
Improving the way time-series data is handled can lead to substantial benefits. Integrate outlier detection into your forecasting methods to enhance prediction accuracy. Commit to continuous improvement in data analysis practices.
Increase Statistical Analysis Precision
Removing outliers from datasets is a critical step to increase the precision of statistical analyses. Outliers can distort the overall findings and lead to incorrect conclusions. By eliminating these extreme values, the analysis becomes more representative of the norm, providing more accurate insights. This ensures that the decisions based on the data are sound and informed.
Taking the time to clean and refine datasets is paramount. Adopt meticulous data cleaning practices to strengthen your analysis results. Regularly review your methodologies to maintain high standards.
Reveal Unusual Customer Behaviors
Identifying outliers in customer data can reveal unusual purchasing behaviors that may otherwise go unnoticed. These anomalies can provide valuable insights into niche markets or specific customer needs. Understanding these unique behaviors allows companies to tailor their marketing strategies and product offerings more effectively. This can lead to increased customer satisfaction and loyalty.
Delving into outlier detection in customer data can unlock new business opportunities. Apply outlier detection techniques in customer analysis to uncover hidden patterns. Stay proactive in exploring unique consumer behaviors.
Pinpoint Unusual Data Patterns
Outlier detection helps pinpoint unusual data patterns, which is crucial for improving decision-making processes. By recognizing these anomalies, businesses can better understand underlying issues or opportunities in their operations. This improved awareness can lead to more strategic and effective decisions. Whether it's in finance, marketing, or operations, enhanced analysis quality contributes to overall business success.
Utilizing outlier detection can thus provide significant advantages. Embrace outlier detection methods to refine your decision-making process. Ensure your organization is leveraging the full potential of data analysis techniques.