What Are Examples of Machine Learning Models that Provide Unexpected Insights?
Data Science Spotlight
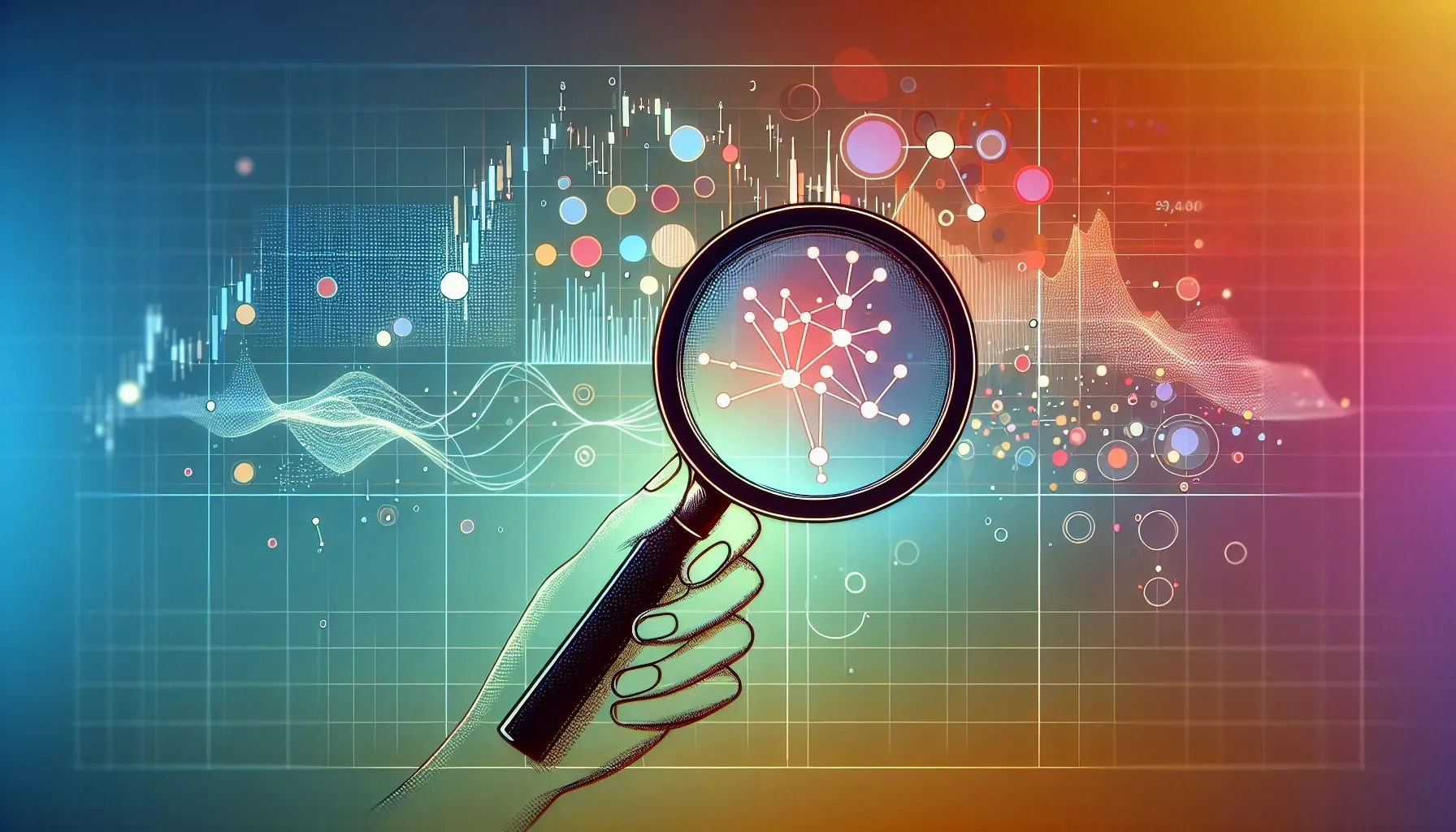
What Are Examples of Machine Learning Models that Provide Unexpected Insights?
In the quest to unearth hidden patterns and insights from complex datasets, we turned to the experts and compiled four diverse perspectives. From a data scientist's clustering model revealing healthcare access issues to a co-founder & CEO's use of linear regression to guide R&D investment strategies, discover how these professionals leveraged machine learning models to achieve unexpected insights.
- Clustering Reveals Healthcare Access Issues
- Deep Learning Unveils Hidden Anomalies
- Random Forest Uncovers Churn Tenure Link
- Linear Regression Guides R&D Investment Strategy
Clustering Reveals Healthcare Access Issues
We used an unsupervised clustering model to better understand patient uptake of a mental health product. Mental health stigma has a big impact on patients' decisions to seek care, so we used publicly available social determinants of health in our clustering to try and identify groups of patients facing different types of stigma. While these factors did impact patient treatment decisions, some groups that faced high stigma still sought treatment at high rates.
It turned out that these groups seemed to have an easier time finding a doctor, while other groups were getting stuck at this stage. It really underscored the importance of easy access to healthcare in patient journeys, and we're hoping to take some steps to make that process even easier.
Deep Learning Unveils Hidden Anomalies
One transformative model that delivered unforeseen insights into our data was the implementation of a deep neural network for anomaly detection. Implementing a deep neural network for anomaly detection revolutionized our understanding of complex datasets, revealing hidden patterns previously elusive to traditional methods. Our methodical deployment began with careful dataset curation, covering diverse features and anomalies. Leveraging cutting-edge deep-learning frameworks, we fine-tuned the model's parameters, ensuring optimal performance.
Crucially, we prioritized proactive model interpretation using techniques like layer-wise relevance propagation and activation maximization. This approach unveiled the rationale behind predictions, enabling us to identify anomalies with unprecedented accuracy.
The results were groundbreaking, as the model not only detected known anomalies but also unearthed previously undiscovered irregularities, guiding further investigation and optimization.
In essence, by harnessing deep learning and adopting a rigorous interpretation approach, we unlocked unexpected insights that drove innovation and enhanced our understanding of our data ecosystem.
Random Forest Uncovers Churn Tenure Link
One machine-learning model that yielded unexpected insights into data is the Random Forest classifier applied to customer churn prediction in a telecommunications company. Initially, the goal was to identify factors influencing customer churn to improve retention strategies. However, during the analysis, the model revealed a previously unnoticed correlation between customer tenure and churn likelihood.
Traditionally, customer tenure was not considered a significant predictor of churn, as it was assumed that longer-tenured customers were more loyal. However, the Random Forest model highlighted that, contrary to this assumption, customers with mid-range tenures actually had the highest likelihood of churning.
This insight challenged our preconceptions and prompted a deeper investigation into the underlying reasons.
To approach this unexpected finding, further exploratory analysis was conducted to understand the behaviors and characteristics of customers with mid-range tenures. This involved segmentation based on usage patterns, demographics, and service subscriptions.
Surprisingly, it was also discovered that this group exhibited signs of dissatisfaction related to service quality and pricing changes, which existing retention efforts needed to address adequately.
Linear Regression Guides R&D Investment Strategy
As a tech leader, the possibilities of machine learning intrigue me. Most recently, we implemented a linear regression model to predict our resource allocation. Its intuition went beyond, pointing out that our investment in R&D is leading to a surge in product success. This insight later popped up as an unexpected blessing. We, therefore, revised our strategy, investing more in innovation, which resulted in improved product performance. Once again, machine learning shed light where we weren't even looking.