How is Reinforcement Learning Used to Solve Complex Problems by Data Scientists?
Data Science Spotlight
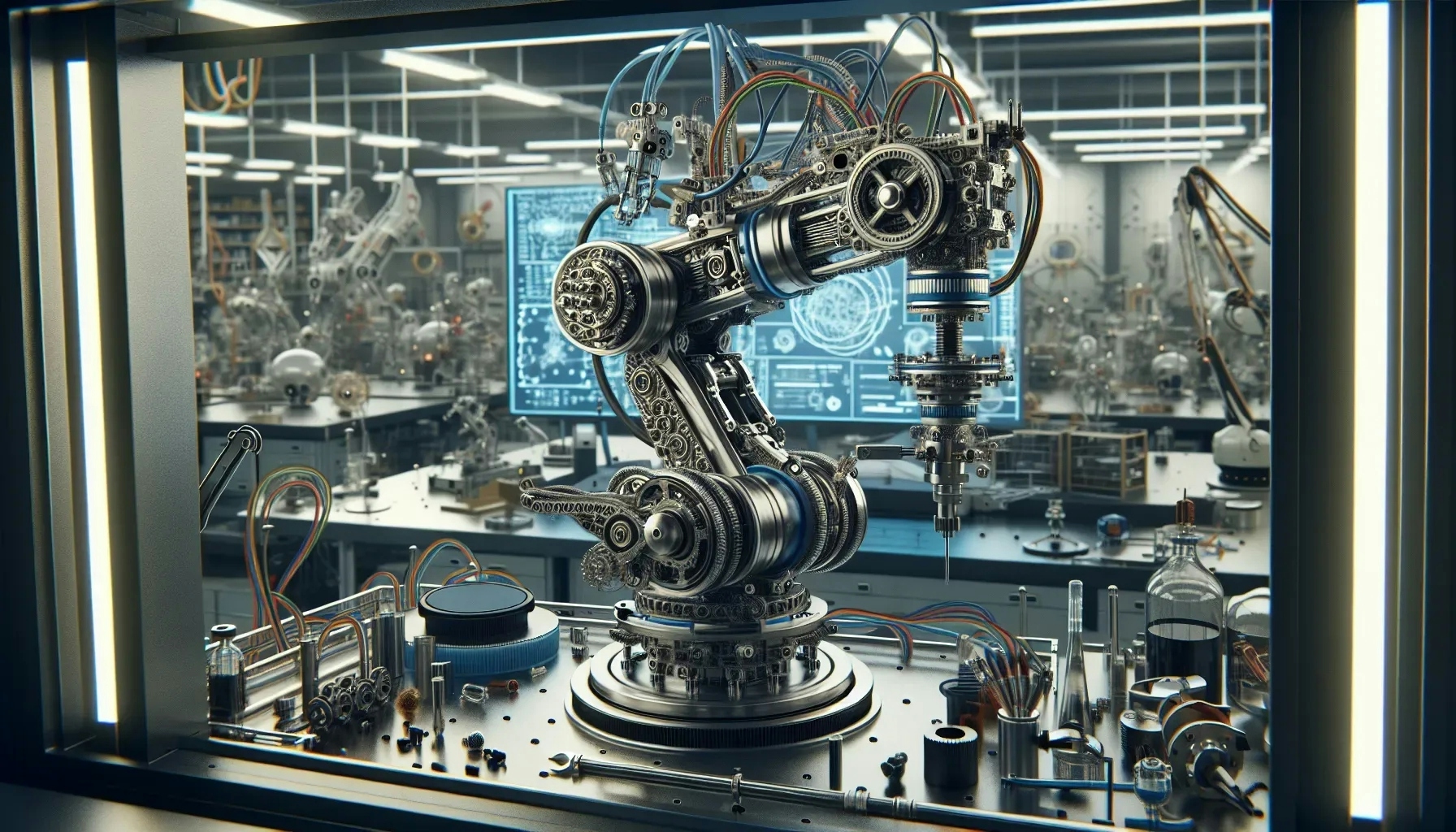
How is Reinforcement Learning Used to Solve Complex Problems by Data Scientists?
From the precise enhancements in automated complaint management systems to the dynamic optimization that adapts in real-time, industry professionals are harnessing the power of reinforcement learning to tackle intricate challenges. A Data Scientist and a Machine Learning Engineer, among others, have shared instances of their success stories. Alongside these expert insights, we also present additional answers that further illustrate the versatility and impact of reinforcement learning in various scenarios.
- Automated Complaint Management Improvement
- Broad Applications of Reinforcement Learning
- Streamlining Problem-Solving with Rewards
- AI Decision-Making in Uncertain Environments
- Personalizing AI Behavior Through Trial and Error
- Enhancing Predictive Accuracy with Reinforcement Learning
- Dynamic Optimization with Real-Time Adaptation
Automated Complaint Management Improvement
For the automation of an online complaint management system for a product-based company, Complaint Codes needed to be predicted based on complainants' inputs—audio transcripts, product descriptions, etc. A model was created based on existing limited data. To improve model efficiency and reach the ultimate goal of removing any human intervention from this step of complaint management, the existing model was retrained every month on new data from that month. This method had the following benefits: 1. Attain higher accuracy using fewer computational resources/time 2. Flexibility to include new variables (apart from complainants' inputs) in subsequent training of the model 3. Retrain the model on-demand depending on data availability 4. Reuse the model for new products, markets.
Broad Applications of Reinforcement Learning
Reinforcement learning (RL) is a powerful machine-learning technique used to solve complex problems across various domains by training agents to make sequential decisions to maximize cumulative rewards. Some notable applications include mastering complex games like Go and Chess, training robots for tasks in robotics, developing algorithms for autonomous vehicles, optimizing trading strategies in finance, enhancing recommendation systems, improving healthcare treatments, and optimizing resource management in logistics and supply chains. RL algorithms enable agents to learn from interaction with environments, making them adaptable to dynamic and uncertain conditions, thus offering promising solutions to a wide range of challenging problems.
Streamlining Problem-Solving with Rewards
Reinforcement learning enables algorithms to refine their actions based upon a system of rewards. The algorithm learns to achieve a goal in an unpredictable environment by understanding which actions yield the highest rewards. This approach mirrors the way humans learn from success and failure, allowing artificial intelligence to evolve without direct human intervention.
As a result, reinforcement learning is particularly effective in situations where manual adjustments are impractical or impossible. Investigate reinforcement learning to see how it could streamline your problem-solving processes.
AI Decision-Making in Uncertain Environments
In a world full of uncertainty, data scientists employ reinforcement learning to aid machines in making decisions without a clear right or wrong answer. The method equips artificial intelligence with the capacity to act and react in real-time circumstances. This autonomy is achieved by exposing the AI to a variety of conditions where it must decide and adapt on its own.
Given enough time and data, these AI systems can navigate complex environments, such as financial markets or robotic maneuvering with greater efficiency. Explore how reinforcement learning can empower your systems to make autonomous decisions in complex environments.
Personalizing AI Behavior Through Trial and Error
The process of teaching AI to act appropriately through reinforcement learning is akin to instructing a child using a system of consequences. By employing a trial-and-error method, the AI experiments with different behaviors and learns which are most beneficial for achieving the desired outcome. This learning process is iterative and allows the AI to adjust its actions in response to changing circumstances.
It is particularly useful for customizing AI behaviors in situations that require a nuanced approach. Consider applying reinforcement learning to refine and personalize your AI's performance.
Enhancing Predictive Accuracy with Reinforcement Learning
Over time, AI models that utilize reinforcement learning become more adept at predicting outcomes and making informed decisions. As the model is exposed to more scenarios, it adjusts and improves its predictive capabilities. This continual refinement is fundamental to developing accurate and reliable AI solutions.
It has widespread applications, from weather forecasting to stock market analysis, where precision and the ability to learn from new data are invaluable. Look into how using reinforcement learning could enhance the predictive accuracy of your data models.
Dynamic Optimization with Real-Time Adaptation
Reinforcement learning stands at the forefront when it comes to solving optimization problems that are dynamic and complex. The approach provides strategies that adapt in real-time, allowing systems to optimize operations even as conditions change. It is ideal for scenarios like routing delivery vehicles in fluctuating traffic or managing energy consumption in smart grids.
By learning from each new challenge, reinforcement learning paves the way toward maximizing efficiency and performance. Take action by considering reinforcement learning for tackling dynamic optimization problems in your field.