7 Ethical Concerns in AI that Deserve More Attention
Data Science Spotlight

7 Ethical Concerns in AI that Deserve More Attention
Delve into the unseen complexities of artificial intelligence as this article sheds light on pressing ethical issues, backed by expert opinions. Discover how biases embedded in algorithms can shape our digital and real-world experiences. Gain essential insights into the AI-driven decisions that impact privacy, accountability, and fairness in technology.
- Algorithmic Bias in AI Systems
- Privacy Concerns with AI Data
- Black-Box AI Accountability Issues
- Systemic Bias in AI Decisions
- Bias in AI Hiring Tools
- AI Bias in Critical Areas
- Addressing AI Bias and Transparency
Algorithmic Bias in AI Systems
One ethical concern related to artificial intelligence that deserves more attention is algorithmic bias. In my experience, biases embedded in AI systems can unintentionally impact decisions in areas like hiring, lending, and even law enforcement. For example, I once worked with a business that struggled to understand why their hiring platform was rejecting diverse candidates. It turned out that the AI had learned patterns from biased training data, filtering out qualified individuals based on factors like gender and education history. This wasn't just unfair—it hurt the business by excluding top talent.
This concern is significant because it can reinforce societal inequalities and have real consequences for individuals. Imagine someone being denied a job or a loan based on their race or background, even when they're fully qualified. These decisions can change lives and reduce access to critical opportunities. The bigger issue is that many organizations using AI don't realize the decision-making process is flawed because it often operates as a "black box." Without transparency, addressing the root of the problem becomes almost impossible.
To tackle this, businesses need to invest in better practices when developing and deploying AI. Training data should reflect diverse perspectives and demographics to avoid perpetuating stereotypes. Explainable AI is another essential step—it allows us to understand how decisions are made and spot issues early. Finally, adopting clear ethical guidelines and working within established regulations ensures accountability. At Parachute, we emphasize responsible technology adoption and urge others to do the same. AI has the potential to be transformative, but only if it's built and used in ways that prioritize fairness.
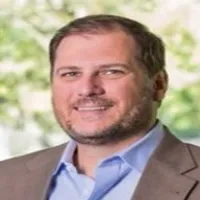
Privacy Concerns with AI Data
The amount of information about ourselves and our businesses that we're willing to give to AI, in particular Large Language Machines such as GPT and Claude, is disturbing.
Not so long ago, there was much concern about the giant sucking noise made by Google and other search engines. However, the practical reality is that traditional search engines were often based on getting to know you just through your search history and behavioral analysis. Of course, this concern hasn't gone away, but the danger has increased by an order of magnitude.
Starting with search itself, it's now well-known that users of AI voice-enabled search give far more away about themselves than with traditional written search. Their search question is longer and their recorded voice can be immediately analyzed for sentiment.
However, the real threat is the number of industry-specific applications being built directly upon Large Language Models. The potential market for this new generation of SaaS type solutions is immense. We see already how it's revolutionizing the domain of marketing content generation. It goes even further in the domain of customer support, with AI Agents accessing entire knowledge bases of organizations and being rigorously "trained" with hundreds of examples of business processes to execute.
On the individual/consumer level, we see the mass adoption of voice assistants well beyond the domain of search (e.g., Alexa, Siri). We also see relatively few if any guardrails as to how users query AI. Large Language Models, for example, aren't designed to say "no" to a child, but rather to generate never-ending output based on what has just been said. Commercially, it's not in the interests of the software vendor to stop the conversation.
AI designs which incorporate "Human in the Loop" feedback are becoming more prevalent, but this is a double-edged sword. HTIL improves the AI output, making it more accurate to the user's requirement. However, it also fine-tunes what the owner of the AI knows about the user or their organization.
As the CEO of an AI software vendor, our approach to solution design has to take into account the digital sovereignty of our customers. As father to a young child, educating our boy not to trust a connected computer or mobile device, while simultaneously learning its potential has become a top parental challenge.
Black-Box AI Accountability Issues
Imagine this: you apply for your dream job, and the decision about whether you're invited for an interview is made entirely by an AI system. Weeks later, you get rejected, but you have no idea why. Was it something in your resume? Was it a glitch in the system? Nobody can explain the decision because even the people who built the AI don't fully understand how it works. This is what happens when we rely on black-box AI models-systems that make decisions in ways that are too complex to explain.
Now, think about accountability. If the decision was unfair-maybe the AI had a bias against certain phrases in your resume-who should you turn to? The company that used the system? The engineers who built it? Or do you just have to accept it and move on? This lack of clarity about who's responsible is a huge problem.
For me, this hits close to home. As the founder of Seekario.ai, I've dedicated my career to building AI tools that simplify and empower the job-seeking process. I've seen firsthand how AI can transform lives by helping people create tailored resumes, improve their profiles, and connect with opportunities. But I've also seen how it can fail when decisions aren't transparent or when accountability is murky. These failures erode trust in the technology and leave people feeling helpless.
The combination of these two issues-AI systems that operate like black boxes and the confusion about accountability-feels like a ticking time bomb. If we don't address them, we risk creating a world where AI makes decisions that shape our lives, yet no one can explain or take responsibility for those decisions. For AI to truly help us, it needs to be something we can question, understand, and trust-not just a mysterious tool we're forced to accept.
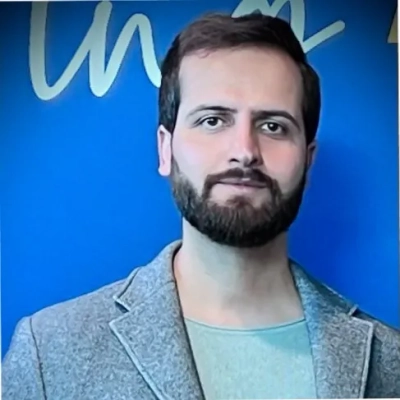
Systemic Bias in AI Decisions
Imagine a world where decisions about hiring, criminal sentencing, or loan approvals are made not by humans, but by algorithms trained on historical data. What if these algorithms, reflecting historical prejudices, perpetuate systemic injustices?
Introduction
Artificial intelligence has changed decision-making across various domains, but its rapid integration into crucial sectors comes with ethical challenges. One such challenge is algorithmic bias, where AI systems adopt the prejudices present in historical data and design, potentially influencing significant outcomes in society. This issue is particularly concerning in high-stakes areas, such as criminal justice, where biased decisions can profoundly affect individual lives and community trust.
Example: Bias in Criminal Justice
In the criminal justice system, risk assessment tools are employed to predict the likelihood of a defendant reoffending. However, studies have shown that these models, often built on historical arrest and sentencing data, may inadvertently overestimate the risk for minority defendants. In one analysis, researchers found that the model's false positive rate for predicting recidivism was 35% higher for minority defendants compared to white defendants. This discrepancy suggests that the data used to train these systems carry the legacy of over-policing and systemic discrimination.
Personal Significance
This concern strikes a personal chord because ensuring fairness in technology is not only a professional responsibility but a moral imperative. As a society, we owe it to every individual to develop AI systems that do not reinforce existing inequalities but rather promote fairness and justice.
Conclusion
Algorithmic bias in AI remains a pressing ethical concern, especially in high-stakes fields like criminal justice. The case study discussed illustrates the tangible impact biased AI systems can have and demonstrates that even targeted interventions can lead to significant improvements in fairness. It is incumbent on us—researchers, developers, and policymakers—to continuously refine our models, ensuring they serve all segments of society without prejudice.
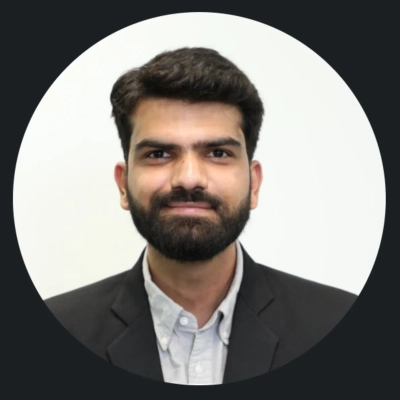
Bias in AI Hiring Tools
One ethical concern with artificial intelligence that I think deserves more attention is the potential for bias in AI systems. If AI is trained on biased data, it can perpetuate or even amplify existing inequalities. This is particularly concerning when AI is used in areas like hiring, law enforcement, or healthcare, where decisions can significantly affect people's lives.
For example, there have been cases where AI hiring tools have unfairly favored male candidates over female candidates simply because the data used to train these systems reflected gender imbalances in past hiring practices. This concern is important to me because AI has the potential to impact so many areas of life, and we need to ensure it's used fairly and responsibly.
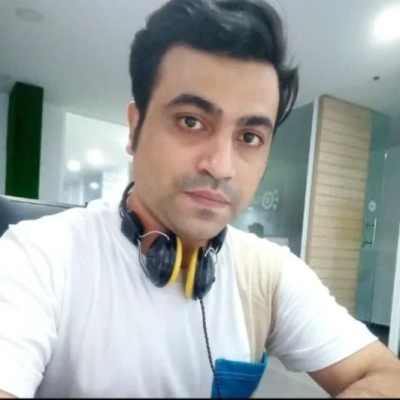
AI Bias in Critical Areas
AI systems often inherit biases from the data they are trained on, leading to unfair or discriminatory outcomes in critical areas such as hiring, lending, law enforcement, and health care. It's like teaching a robot to cook and realizing it only makes your least favorite dish over and over. It is concerning as it impacts real lives, perpetuating societal inequalities under the guise of objectivity. AI is becoming more ingrained in decision-making, and addressing bias is a technical challenge and a moral imperative to ensure fairness and inclusivity. If we're building the future, let's make sure it's fair and not just for those who look like the dataset.
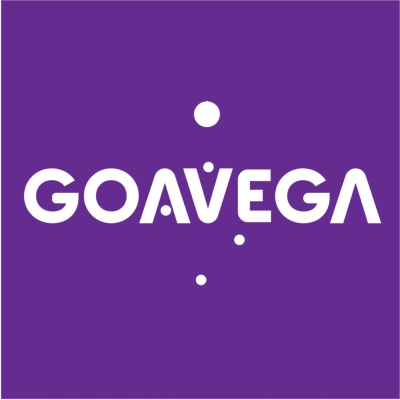
Addressing AI Bias and Transparency
One ethical concern related to artificial intelligence that deserves more attention is algorithmic bias. AI systems are often trained on historical data, which can inadvertently encode and amplify existing societal biases. This is significant because these biases can perpetuate inequality, especially in critical areas like hiring, lending, or healthcare decision-making.
What makes this issue even more pressing is the lack of transparency in how many AI models arrive at their decisions. Without understanding the "why" behind an AI's output, it's nearly impossible to address unfairness effectively. For me, this hits home because AI has incredible potential to level the playing field, but unchecked bias can do the opposite, reinforcing systemic issues instead of solving them. We need to prioritize diverse datasets and rigorous audits to ensure AI works equitably for everyone.
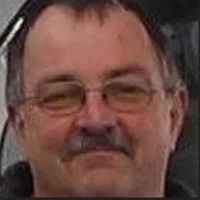