6 Insights from Nlp On Analyzing Social Media Data
Unlock the secrets of social media dynamics through the lens of Natural Language Processing (NLP). This article delves into expert insights on how data analysis can influence trends, shape logistics, and even track public sentiment on pressing issues. Explore the profound impact of NLP-driven social media analysis on everything from marketing strategies to policy-making.
- Genuine Tweets Go Viral
- Optimize Logistics Based on Sentiment Analysis
- Identify Influencers and Improve Product Features
- Track Sentiment on Lockdown Policies
- Analyze Fan Reactions to PPV Model
- Real-Time Social Media Analysis
Genuine Tweets Go Viral
I looked at thousands of tweets using NLP and discovered that the ones that went viral weren't overly polished—they felt genuine. Casual, unfiltered language outperformed anything that sounded scripted.
The main takeaway is that movement encourages sharing. Tweets that elicited laughter, agreement, or immediate reactions spread the quickest. A simple emoji or a relatable phrase could really change the game.
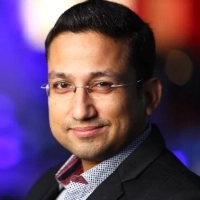
Optimize Logistics Based on Sentiment Analysis
Using NLP to analyze social media data was a game-changer in one of my ecommerce brand's campaigns. We wanted to understand how customers felt about our product beyond basic engagement metrics, so we used NLP sentiment analysis to break down thousands of customer comments and reviews. Instead of guessing, we got clear insights into what customers loved, what frustrated them, and recurring themes in feedback.
One major finding was that while customers loved the product itself, many complained about slow shipping times. This insight pushed us to optimize our logistics and improve our messaging around delivery expectations, reducing negative feedback by over 30% in the following months. NLP made it possible to spot patterns at scale, helping us refine our marketing, enhance the customer experience, and boost retention—all based on real, unfiltered customer sentiment.
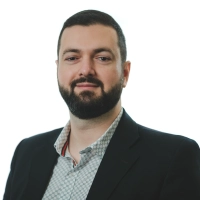
Identify Influencers and Improve Product Features
I once led a project at a major tech company where we applied NLP to analyze massive volumes of social media posts-specifically, users discussing our newly launched product features. We collected mentions from platforms like Twitter and Instagram, then used a combination of rule-based filtering and machine learning algorithms to classify the posts by sentiment, topic, and user influence level.
One of the first steps was cleaning and normalizing the text. We removed usernames, URLs, and common stop words, then employed techniques like tokenization and lemmatization to transform the data into a more analyzable format. From there, we applied a sentiment analysis model to assign positive, negative, or neutral scores to each post, and we also used topic modeling (with tools such as LDA) to cluster similar discussions. This helped us understand not just how people felt about the product, but also which specific features or pain points were trending at any given moment.
The insights we gleaned were both quantitative and qualitative. On the quantitative side, we noticed a spike in negative sentiment every time a certain feature failed to work smoothly on a popular mobile device. That gave our engineering team a clear signal to investigate compatibility issues on that specific device model. On the qualitative side, the topic modeling revealed that users were consistently praising one feature we hadn't even prioritized in the product roadmap-leading us to double down on its development. We also identified the biggest influencers driving the conversation; by focusing engagement efforts on just a handful of highly active users, we saw a ripple effect in overall sentiment and awareness.
In the end, this project demonstrated how powerful NLP can be for quickly synthesizing thousands or even millions of social media posts into actionable insights. It saved countless hours of manual data review, gave our product managers empirical evidence about which features needed attention, and helped marketing teams target high-impact user groups. More importantly, it underscored how user feedback in the social media space can be a goldmine if you have the right tools and processes to interpret it effectively.
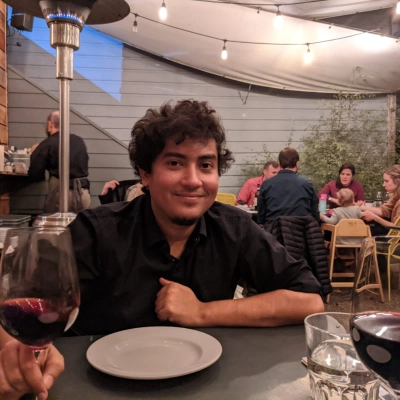
Track Sentiment on Lockdown Policies
During the COVID-19 pandemic, I worked on an NLP-driven analysis of social media sentiment regarding lockdown policies. Governments worldwide faced the challenge of balancing public health and economic stability, and understanding real-time public sentiment was critical for effective policy decisions. Our goal was to quantify these sentiments by analyzing large-scale Twitter and Reddit data, capturing shifts in public opinion as lockdown measures evolved.
We began by collecting and preprocessing data, filtering tweets and posts using relevant keywords such as "lockdown," "quarantine," and "stay-at-home orders." We implemented Named Entity Recognition (NER) to identify references to specific policies and locations, ensuring a more granular analysis. Sentiment analysis was performed using a fine-tuned BERT model, which outperformed traditional lexicon-based approaches like VADER in capturing context-dependent expressions. This allowed us to classify posts as positive, neutral, or negative, providing a nuanced understanding of public sentiment dynamics.
Beyond sentiment classification, we employed topic modeling techniques such as Latent Dirichlet Allocation (LDA) to identify recurring themes in discussions. Key topics included mental health struggles, economic concerns, and frustrations with inconsistent government communication. We also observed spikes in misinformation, particularly conspiracy theories about government control, which correlated with increased lockdown resistance. Temporal analysis revealed that public sentiment fluctuated significantly in response to major events, such as rising COVID-19 cases or government relief announcements.
One interesting finding was how sentiment shifted over time within the same groups. Early in the pandemic, there was broad support for lockdowns, with many posts emphasizing community responsibility and public health. However, as months passed, frustration grew, especially among small business owners, gig workers, and parents juggling remote work with child care. This shift was reflected in language patterns, where words associated with "sacrifice" and "safety" in early months gradually gave way to terms like "fatigue," "burnout," and "unfair" as lockdowns persisted. We also found that sentiment decay was faster in regions with unclear or frequently changing government policies, indicating that uncertainty played a major role in public dissatisfaction.
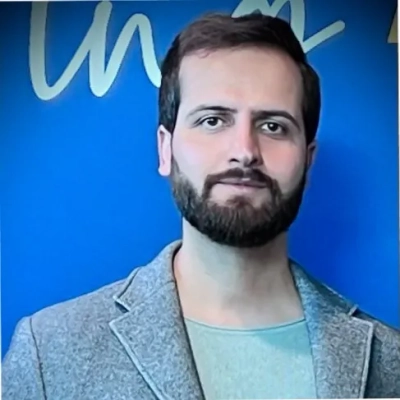
Analyze Fan Reactions to PPV Model
I used NLP as a data analytics consultant when working with a client from a news company. As part of our project, we extracted 5000 tweets from the Twitter API and created the analysis in R.
The project was aimed at analyzing the reactions of English Premier League fans towards a new controversial PPV model which made it more expensive to watch soccer games.
We found some words that were frequently mentioned by the fans on Twitter such as:
Scrap - calling English Premier League to scrap the PPV model
Boycottppvlive - a popular hashtag used by the fans
High Cost - fans expressed their main concern with the model
We then analyzed the distribution of the tweets by sentiment score. We found that:
There was a noticeable percentage of people with highly negative sentiments but the overall analysis showed the normal distribution of sentiment towards the PPV model.
There was a huge increase in negative sentiment when the price of PPV was revised
Aston Villa, Arsenal, and Chelsea fans had the lowest sentiment score of their tweets highlighting their dissatisfaction
The fans from Leeds, North London, and Wales were most dissatisfied with the PPV model.
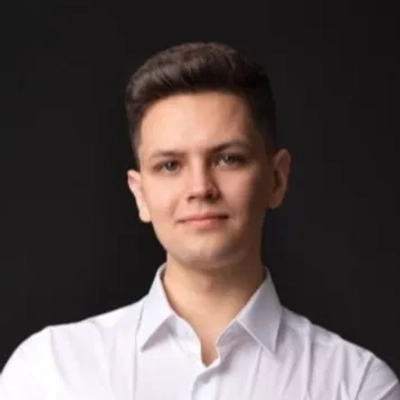
Real-Time Social Media Analysis
Hi!
Last year we partnered with KWatch.io in order to analyze social media data in real-time with advanced NLP techniques. Our goal was to analyze Reddit, and Twitter in real-time. Here are our key takeaways:
- Real-time analysis works great if you focus on small and efficient NLP models (like spaCy for example, or small Transformer-based models like DistilBERT)
- You need to focus on use cases that are not too demanding, like sentiment analysis, text classification, or intent detection
- NLP is not necessarily the hardest part. Reliably plugging into the social media live streams of data and ingesting the data reliably in real-time can be very challenging.
Please don't hesitate to ask me more questions!
Best,
Julien
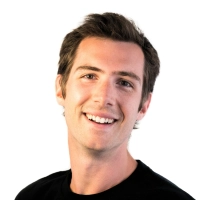