6 Ethical Considerations Critical to Machine Learning
Data Science Spotlight

6 Ethical Considerations Critical to Machine Learning
Delve into the crucial ethical considerations that are pivotal to the advancement of machine learning. This article covers insights from leading experts in the field, ensuring you receive well-rounded and authoritative perspectives. Uncover the importance of reliability, transparency, bias management, data privacy, and more.
- Prioritize Language Model Reliability
- Ensure Transparency In AI Decisions
- Anticipate Unintended Consequences
- Manage Bias In AI Algorithms
- Prioritize Data Privacy In Tech
- Consider Unintentional Marginalization
Prioritize Language Model Reliability
One ethical consideration with AI that really needs more focus is the reliability of language models, especially when it comes to science and math. As a scientist and science communicator, I've noticed that these models often give incorrect answers or omit important aspects of a scientific concept. In some cases, this is because they rely on outdated or incomplete information. It can also happen because language models may not handle the rigorous logic required in these fields. Despite these shortcomings, language models almost always portray confidence, which can lead to a false sense of trust in their outputs and potentially spread misinformation.
In my work as a science communicator, I address this by always cross-checking the results against reliable, up-to-date sources from subject matter experts and peer-reviewed journals. I always tell people, "never trust a language learning model for math or science." To use language models efficiently and accurately, the first step is to understand what these tools are meant for, and know their limitations. You wouldn't use a wrench to tighten a screw, so don't use a language model for math or scientific reasoning.
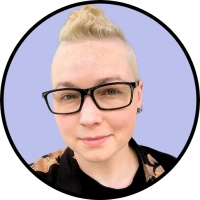
Ensure Transparency In AI Decisions
One ethical consideration in machine learning that deserves more attention is transparency in AI decision-making. Many AI systems operate like a 'black box,' meaning their decision-making process isn't clear. This lack of transparency makes it hard to understand why certain choices are made, especially when they impact people's lives. At Tech Advisors, we've seen businesses struggle with AI-driven security tools that flag legitimate activities as threats without clear reasoning. If users can't see why a system made a decision, they can't correct mistakes or improve outcomes.
A major concern is when AI models are trained on biased data, leading to unfair decisions. I remember discussing this with Elmo Taddeo from Parachute. He shared how a financial company he worked with faced issues when their AI-powered fraud detection system disproportionately flagged certain customer demographics. Without proper oversight, biased models can reinforce discrimination. The best way to prevent this is to train AI on diverse, high-quality data and continuously audit its performance. Explainable AI (XAI) also helps by making AI decisions more understandable and accountable.
At Tech Advisors, we emphasize the importance of human oversight in AI-driven security and compliance tools. AI should assist, not replace, human judgment. When working with clients, we ensure they have the ability to review AI-generated decisions and make adjustments when needed. If an AI system flags a cybersecurity risk, for example, IT teams should have clear visibility into why that happened. Transparency builds trust and ensures AI benefits businesses without creating unnecessary risks.
Anticipate Unintended Consequences
One crucial ethical consideration is anticipating unintended consequences—especially how a technology could be misused or cause harm beyond its intended scope.
Take facial recognition tech. It was developed for security, but in practice, it has been used to target marginalized communities, leading to wrongful arrests and privacy violations.
As an engineer, you can't just think about what a system can do, but also who might abuse it and how. When Amazon's Rekognition tool was found to have racial biases, it sparked backlash. This should've been caught in the development phase with diverse data sets and stronger testing.
Engineers need to push for more rigorous ethical testing before deploying technology into the wild. If you only consider functionality and ignore potential social impact, you're setting up a minefield for misuse.
Ethics should be as integral to the development process as performance metrics.
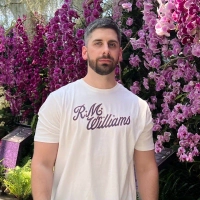
Manage Bias In AI Algorithms
One unexpected challenge I faced when implementing AI in HR was managing bias in the algorithms. Even though AI is often seen as neutral, I quickly realized that it can unintentionally replicate human biases if the data it's trained on is flawed. To overcome this, we put a lot of effort into auditing our datasets and testing the AI systems rigorously before deploying them. We also made sure to involve diverse teams in the process to catch blind spots.
For others facing this challenge, I'd recommend starting with a thorough review of your data and involving different perspectives early on. Don't assume the AI will be perfect—keep testing and refining it continuously to ensure fairness and accuracy in decision-making.
Prioritize Data Privacy In Tech
One crucial ethical consideration in developing or deploying new technologies is data privacy. As tech experts and engineers, we must prioritize the protection of user data and ensure transparency in how it is collected, used, and shared. A specific example illustrating this point is the controversy surrounding facial recognition technology. Many companies have developed this technology for various applications, from security to user authentication. However, the potential for misuse, such as mass surveillance and racial profiling, raises significant ethical concerns.
For instance, in 2020, cities like San Francisco banned the use of facial recognition technology by government agencies due to concerns over privacy violations and biased outcomes. This decision highlighted the importance of considering not only the technological capabilities but also the societal implications of deploying such systems.
As developers, it is essential to integrate robust data privacy measures from the outset, including obtaining informed consent from users and implementing strong encryption protocols. Establishing ethical guidelines and continuously engaging with stakeholders, including users and advocacy groups, can help ensure that new technologies are designed and deployed in a manner that respects individual rights and fosters public trust. By prioritizing ethical considerations like data privacy, we can develop technologies that contribute positively to society while mitigating potential harms.
Consider Unintentional Marginalization
One ethical consideration I always think about when developing or deploying new technologies is how they might unintentionally marginalize or exclude certain groups. It's something that can get lost in the excitement of innovation, but the truth is, technology doesn't operate in a vacuum. It interacts with the messy, diverse realities of people's lives. And when we overlook this, the impact can be pretty harmful.
Take AI-driven hiring tools, for example. They're supposed to make the process more efficient and objective, but if the algorithms are trained on biased data, they can end up reinforcing existing inequalities. Think about a tool that's meant to screen job applicants faster. If it's built on data that reflects historical biases such as favoring candidates from certain schools or backgrounds, it can end up shutting out highly qualified people who just don't fit that mold. You've got a piece of tech that's meant to improve fairness, but in practice, it's doing the exact opposite, making it even harder for some folks to get a foot in the door.
This is why I believe that it's not enough to just test a technology for how well it functions technically. You've got to ask questions like, "Who is this working for, and who might it be working against?" Even something as simple as designing a website can have this problem. If you don't consider people with disabilities, like those who use screen readers, you're basically building a barrier for them, even if unintentionally. And the same goes for AI systems or automated processes. It's about understanding the impact on everyone who might come into contact with the technology, not just the intended user.
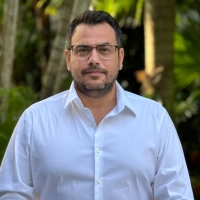