4 Areas Where Nlp Falls Short of Human Abilities
Data Science Spotlight

4 Areas Where Nlp Falls Short of Human Abilities
Discover the limitations of Natural Language Processing (NLP) through an expert lens in this insightful exploration. Uncover why machines struggle to grasp complex logical reasoning, miss subtle contextual cues in human communication, and lack nuanced emotional understanding. This article delves into the critical areas where AI has not yet matched human common sense, despite advancements in technology.
- NLP Struggles with Multi-Step Logical Reasoning
- AI Misses Subtle Context in Human Language
- NLP Lacks Nuanced Emotional Understanding
- AI Falls Short in Common Sense Reasoning
NLP Struggles with Multi-Step Logical Reasoning
From my experience, NLP still falls short of human capabilities in multi-step logical reasoning. I will explain why I think so from several aspects.
When solving math word problems, an NLP model can sometimes correctly perform a certain local derivation. However, it often fails to seamlessly connect all the derivation steps, which ultimately leads to an incorrect conclusion. The reason behind this is that during the reasoning process, humans establish a clear causal chain, ensuring that each derivation step is grounded in the preceding information. In contrast, an NLP model may lose crucial information, thus drawing incorrect conclusions. Moreover, NLP lacks the ability that humans possess to check their conclusions, identify potential errors, and correct them. When the model makes mistakes at a certain stage, it usually fails to recognize its error in the derivation and instead continues to proceed along the wrong path, eventually arriving at an incorrect or unreasonable answer.
Similarly, in reading comprehension tasks, if a question requires referring to the information presented at the beginning of an article, and the model has "forgotten" these details by the time it processes the later part of the text, the reasoning will go awry. This is because multi-step reasoning generally involves the understanding and integration of long texts or multiple premises. Currently, though the Transformer architecture uses the self-attention mechanism to capture long-range dependencies, when the reasoning chain exceeds a certain length, the model is likely to overlook the key information derived earlier.
To enhance the multi-step reasoning ability of NLP, two main improvements are needed. First, it's essential to improve the memory mechanism of the model so that it can retain key information during the reasoning process. Second, a self-correction mechanism should be developed for the model. This would enable the model to detect and correct its errors during the reasoning process, thereby enhancing the reliability of the reasoning chain.
AI Misses Subtle Context in Human Language
Natural language processing still struggles with understanding context and nuance like humans do. It's one thing to process words; it's another to truly grasp what's behind them. Sarcasm, humor, cultural references--those can throw NLP models off big time. During a UGC campaign for Amazon, I tested AI-generated captions for videos. They were technically accurate but missed the playful tone needed for the brand's voice. It's not just about understanding words; it's about catching the vibe.
The biggest challenge is teaching models to pick up on those subtleties. Even advanced models still struggle with context-switching and understanding the emotional undertone behind user-generated content. Improving this would mean training models on diverse datasets that include informal speech, slang, and cultural nuances. It's a tough one, but it's where the real progress needs to happen.
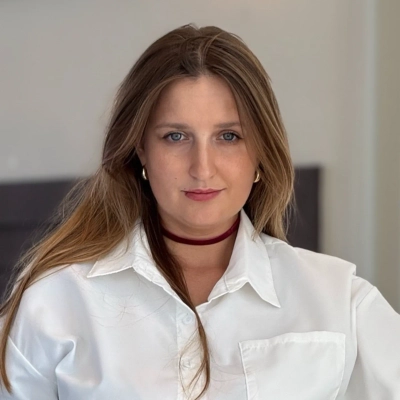
NLP Lacks Nuanced Emotional Understanding
One area where Natural Language Processing (NLP) still falls short is understanding context and nuance in human emotions. While NLP models like ChatGPT have made significant progress in sentiment analysis, they often struggle with sarcasm, irony, and cultural references. For example, a sentence like "Oh great, another Monday" could be interpreted as positive when, in reality, it's sarcasm.
Challenges to Address:
Emotional Intelligence - NLP needs to better detect subtle emotional cues in text.
Context Awareness - Words change meaning based on context, and models still lack deep contextual understanding like humans.
Ethical Bias - NLP models can unintentionally reflect biases present in training data, leading to inaccurate or unfair responses.
To bridge this gap, advancements in multimodal AI (text + visual + audio) and more diverse datasets will be key to improving NLP's human-like comprehension.
For more insights on AI advancements, check out https://teksacademy.com/courses/best-data-science-course-training-institute
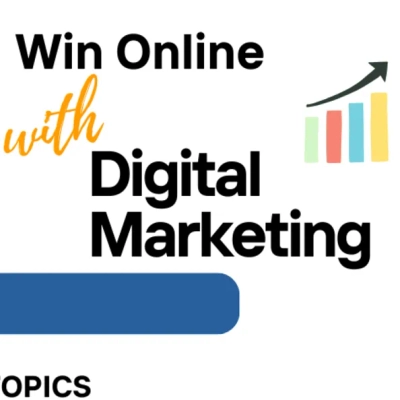
AI Falls Short in Common Sense Reasoning
One of the top limitations of artificial intelligence is the lack of common sense and deep understanding of context. AI systems, even advanced ones like GPT-4, largely rely on pattern recognition and statistical associations derived from vast amounts of data. As a result, they often lack the ability to reason like humans and struggle with tasks that require deep understanding or complex decision-making.
AI falls short in understanding:
- context and ambiguity
- creativity and innovation
- emotional intelligence
- morality and ethics
- adaptability and generalization
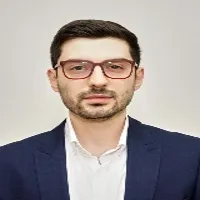