Tips for Aspiring Data Analysts
Data Science Spotlight
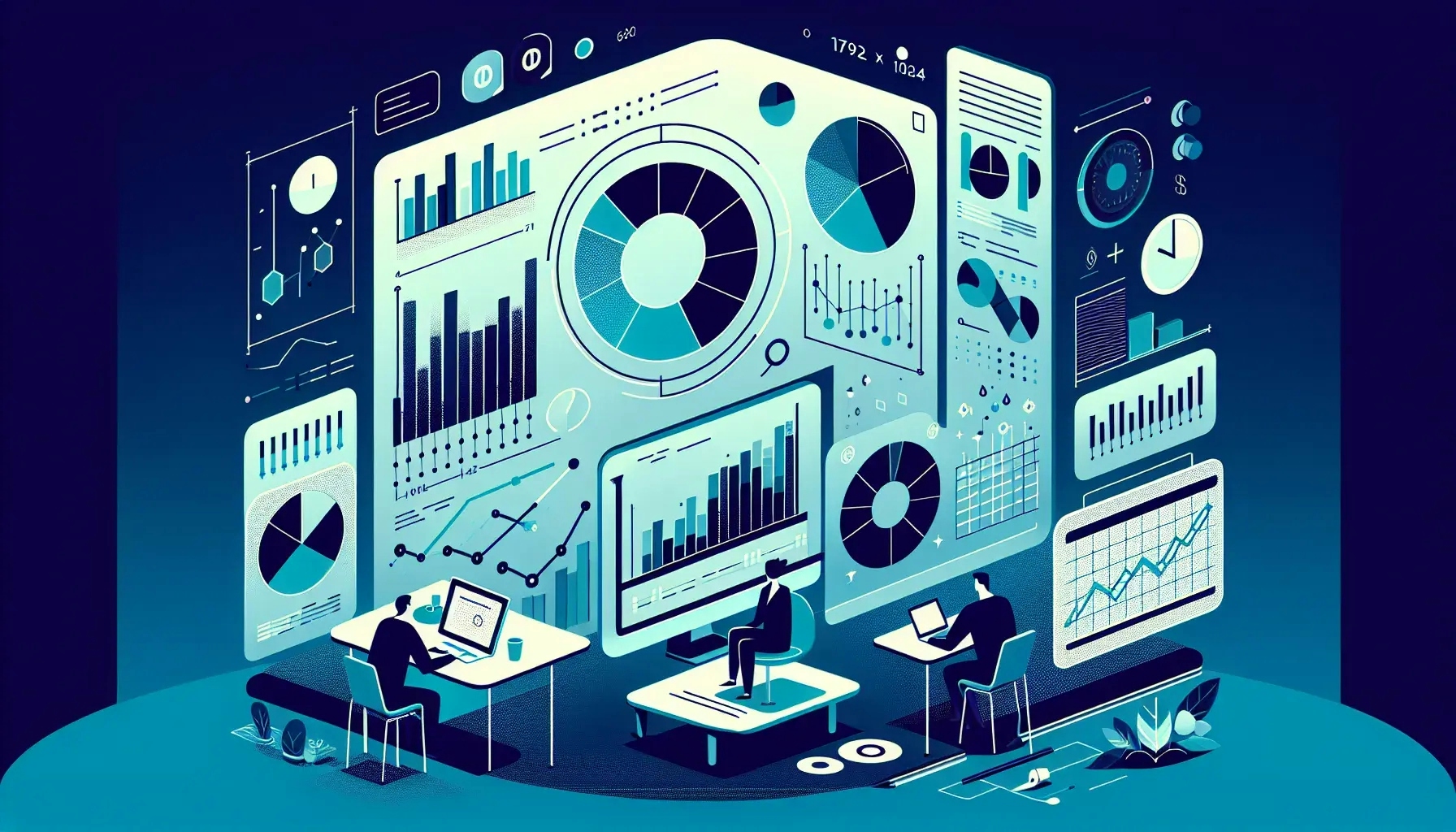
Data analysis is a rapidly growing field, with a wealth of opportunities for those who are ready to dive in. This blog post provides a comprehensive guide for those who aspire to become data analysts. It covers everything from the necessary skills and qualifications to the best practices in the industry.
Understanding the Role of a Data Analyst
A data analyst's role is multifaceted and requires a unique blend of skills. They are responsible for collecting, processing, and performing statistical analyses of data. Their insights guide strategic decisions and help organizations make data-driven decisions.
Data analysts work in various sectors, including healthcare, finance, marketing, and technology. Regardless of the industry, their primary role remains the same: to interpret complex data and turn it into understandable, actionable information.
To succeed as a data analyst, you need to develop a strong foundation in mathematics and statistics. You should also have a good understanding of data structures and databases. Familiarity with programming languages such as Python or R is also beneficial.
Acquiring the Necessary Skills and Qualifications
While a degree in mathematics, statistics, or a related field is often required for data analyst roles, it's not the only path to success. Many data analysts come from diverse backgrounds and have used online courses, boot camps, or self-study to acquire the necessary skills.
In addition to technical skills, data analysts need to develop strong problem-solving abilities. They often encounter complex data sets and must find innovative ways to extract meaningful insights.
Communication skills are also crucial. Data analysts must be able to explain their findings to non-technical team members and stakeholders. They should be able to create clear, compelling reports and visualizations that effectively communicate their insights.
Gaining Practical Experience
Practical experience is invaluable for aspiring data analysts. Internships, part-time jobs, or volunteer work can provide hands-on experience with real-world data. These opportunities also allow you to apply what you've learned in a practical setting.
Working on personal projects is another excellent way to gain experience. You could analyze a dataset that interests you, such as sports statistics or social media data. This not only helps you develop your skills but also allows you to create a portfolio of work to show potential employers.
Staying Current with Industry Trends
The field of data analysis is continually evolving. New tools and techniques are regularly introduced, and staying current is essential for success.
Regularly reading industry publications, attending webinars, and participating in online forums can help you stay up-to-date. Joining professional organizations can also provide networking opportunities and access to resources.
Cultivating a Growth Mindset
A growth mindset is crucial in the ever-evolving field of data analysis. This means being open to learning, willing to take on challenges, and not being afraid to make mistakes.
Data analysts should continually seek to improve their skills and knowledge. This could involve learning a new programming language, mastering a new data analysis tool, or delving deeper into a specific area of statistics.
Building a Strong Professional Network
Networking is often overlooked but can be incredibly beneficial for aspiring data analysts. It can lead to job opportunities, mentorship, and collaboration.
You can start building your network by attending industry events and joining online communities. Don't be afraid to reach out to professionals in the field. Most people are happy to share their experiences and provide advice.
Wrapping Up: Your Journey to Becoming a Data Analyst
Embarking on a career as a data analyst is an exciting journey. It requires a mix of technical skills, practical experience, and a commitment to continuous learning. By following the advice in this post, you'll be well on your way to a successful career in data analysis.