The Ethics of Data Science
Data Science Spotlight
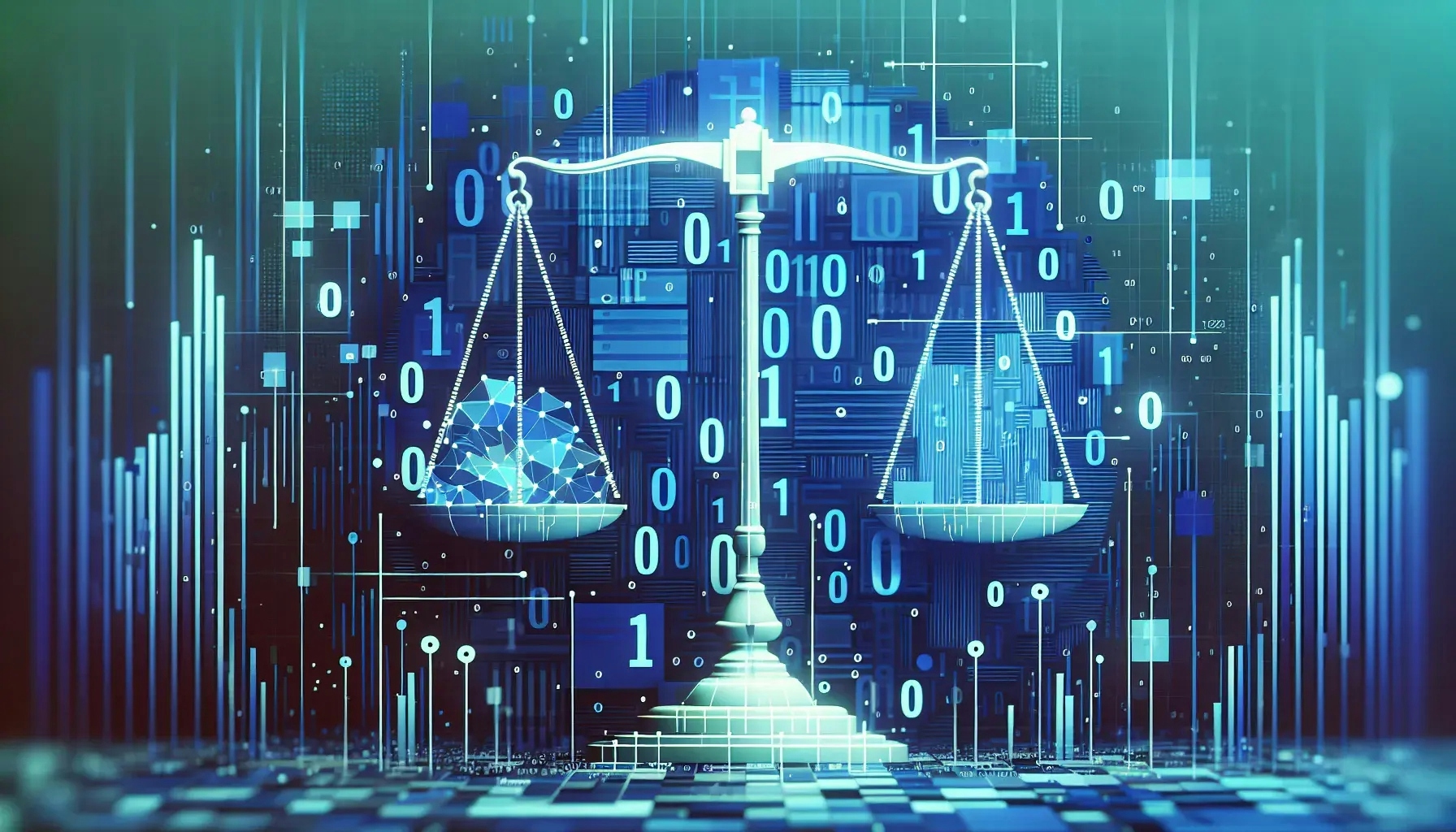
In the rapidly evolving world of technology, data science has emerged as a significant player. It's a field that combines statistics, data analysis, machine learning, and related methods to understand and analyze actual phenomena. However, as with any powerful tool, it comes with its own set of ethical challenges. This blog post aims to explore these challenges and provide insights into the ethical considerations that data scientists must navigate in their work.
The Power and Potential of Data Science
Data science has the potential to revolutionize the way we live, work, and think. It can help us make sense of the vast amounts of data we generate every day. From predicting consumer behavior to improving healthcare outcomes, data science can provide valuable insights and solutions to complex problems.
However, the power of data science also brings with it a host of ethical considerations. As data scientists, we must be aware of these challenges and strive to navigate them responsibly. We must ensure that our work does not harm individuals or communities, and that it respects the principles of fairness, transparency, and accountability.
Ethical Challenges in Data Science
The ethical challenges in data science are numerous and varied. They range from issues of privacy and consent to questions of bias and discrimination. For example, how do we ensure that the data we collect is used responsibly and does not infringe on individuals' privacy? How do we ensure that our algorithms do not perpetuate harmful biases or lead to unfair outcomes?
These are not easy questions to answer, and they require a deep understanding of both the technical aspects of data science and the ethical principles that guide our work. As data scientists, we must be committed to continuous learning and reflection in order to navigate these challenges effectively.
Privacy and Consent in Data Science
One of the most pressing ethical issues in data science is that of privacy and consent. With the increasing availability of personal data, it is crucial that we respect individuals' rights to control how their data is used.
This means ensuring that we obtain informed consent before collecting or using personal data. It also means being transparent about how we use this data and taking steps to protect it from misuse or unauthorized access.
However, privacy and consent are not always straightforward issues. For example, in the age of big data, it can be difficult to obtain informed consent from every individual whose data we collect. Similarly, it can be challenging to ensure that individuals understand how their data will be used, especially when dealing with complex algorithms or predictive models.
Bias and Fairness in Data Science
Another significant ethical challenge in data science is that of bias and fairness. Algorithms and predictive models are only as good as the data they are trained on. If this data is biased, the resulting predictions or decisions can also be biased.
For example, if a predictive model is trained on data that includes racial or gender biases, it may perpetuate these biases in its predictions. This can lead to unfair outcomes, such as discrimination in hiring or lending practices.
As data scientists, we must be vigilant in identifying and addressing biases in our data and algorithms. This requires a commitment to fairness and equality, as well as a willingness to question our assumptions and challenge the status quo.
Transparency and Accountability in Data Science
Transparency and accountability are also key ethical considerations in data science. As data scientists, we must be transparent about our methods and assumptions, and we must be accountable for the outcomes of our work.
This means being open about the limitations of our models and the potential risks associated with their use. It also means taking responsibility when our models lead to harmful or unintended outcomes.
However, transparency and accountability can be challenging in the context of complex algorithms or proprietary models. In these cases, it is crucial that we find ways to communicate our methods and findings in a clear and accessible way, and that we establish mechanisms for accountability and oversight.
The Future of Ethics in Data Science
As the field of data science continues to evolve, so too will the ethical challenges we face. New technologies and methods will bring new opportunities, but also new risks and uncertainties.
As data scientists, we must be prepared to navigate these changes and to continually reassess our ethical responsibilities. We must be committed to learning and growth, and to the ongoing development of ethical standards and practices in our field.
The future of ethics in data science is not a fixed destination, but a journey. It is a journey that requires courage, humility, and a deep commitment to the values of fairness, transparency, and accountability.
Concluding Thoughts on the Ethics of Data Science
The ethical landscape of data science is complex and challenging, but it is also a landscape of immense potential. By engaging with these challenges and striving to navigate them responsibly, we can harness the power of data science for the benefit of all. As we move forward, let us do so with a deep commitment to ethics, a spirit of curiosity, and a dedication to making the world a better place.